Can AI be fair?
In the past, most engineers were male. Therefore, engineers must always be male. This – or in a similar way – is how a self-learning system draws conclusions. In German texts it will spit out the generic masculine to describe engineering tasks, or it will suggest to fill vacancies in engineering with male applicants.
Studying the ‘brain’ of AI
Complex systems such as ChatGPT learn from large amounts of data. Their decisions are based on patterns that are reflected in parameters. We are talking about neural networks, explains computer linguist Dagmar Gromann, "Large language models are inspired by how the human brain works. This allows the model to learn patterns – from huge datasets that pass through the network again and again."
And this is the problem: If an AI model is sexist, it has learned to be so from sexist data that we have generated for decades. And the models do not only show bias in terms of gender, but also "with regard to race or ethnicity and pretty much everything else," says Dagmar Gromann. Together with a team at the Centre for Translation Studies, she has studied how smart systems extract information. The research team fed textual data from various disciplines into machine translation systems to analyse how they implicitly learn relations. For English, for example, a clear relationship between ‘criminal’ and ‘black’ was established – even if the texts did not mention ‘black criminals’ (further information about the project is available on the project website).

AI: A fuzzy concept
Artificial intelligence is not a clearly definable technology, but rather a range of different systems and infrastructures. Even if the umbrella term is used in this article, different systems are meant depending on the example. More AI knowledge © iStock
These implicit relations become relevant, for example, when artificial intelligence is used to decide whether applicants receive a loan – or not. When making decisions, certain parameters are protected by the General Data Protection Regulation (at least in Europe). For example, the systems may not use medical data, political opinions, affiliations to trade unions, sexual orientation or colour, explains Erich Prem, computer scientist and philosopher at the University of Vienna, "But even if my input criteria do not include 'colour', the information might be contained in other parameters, such as in a combination of district and occupation, and the system thus still discriminates against a specific group of the population."
No transparency, no charge
"There are countless other examples that show that already marginalised groups are the ones that usually experience discrimination in automated processes," adds Katja Mayer from the Department of Science and Technology Studies. In general, with or without AI, the principle of equal treatment applies in Austria by law. This means: Affected persons can defend themselves against discrimination by artificial intelligence, for example by contacting offices responsible for equal treatment.
However, it is often not exactly easy to ‘prove’ that an AI-powered technology is discriminating against certain groups of people. The AI can justify any of its decisions in page-long statistical formulas, but these explanations are rarely transparent, especially not for its users, explains Mayer.
When AI systems decide
Do we really want systems like this to take autonomous decisions? The fact is that they are already doing so in many areas. For example, in the case of self-driving cars, news aggregators, lethal autonomous weapons in war, or language models explaining the world to us, says Prem, who is an expert on AI ethics. In the tradition of digital humanism, he and his colleagues from the Techphil Group at the Department of Philosophy are exploring the question of how we want to live in a digitised world. Spoiler: Although we are already right in the middle of it, there are no clear answers to this question.
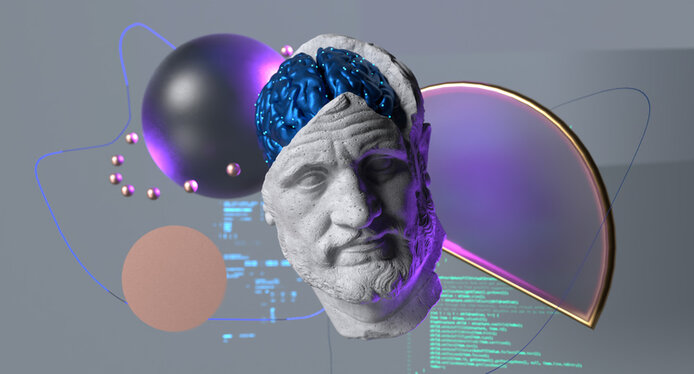
Digital humanism in a nutshell
Digital humanism is an approach that places human values and needs at the heart of digital transformation. The focus is on a human-centred approach, ethics and responsibility, inclusion and accessibility, education and empowerment as well as sustainability and environmental protection. The lecture series ‘Artificial intelligence: areas of tension, challenges and opportunities’, organised by the Department of Contemporary History of the University of Vienna in cooperation with the City of Vienna, dedicated an entire unit to digital humanism. © iStock
What is actually fair?
To give a real-life example: Women are still less likely to receive funding commitments than men. If we want to establish a ‘fair’ AI-powered system that supports the selection process by means of an automated procedure and gives all people equal access to funding, this is quickly formulated, but it is complicated to get to the point or to technically implement it. Fair could mean that the approval rate is the same for men and women. But it could also mean that the rejection rate is the same or that a person who is read as female must always be treated the same as a person who is read as male – regardless of the circumstances. Which of these different interpretations of fairness we want to apply is a political question, says Prem.
Shaping social conditions
But what is fair in a specific situation is usually determined by developers in the design process – due to a lack of political guidelines: "Computer scientists do not just solve technical problems, but shape social conditions to an extent that they would never have expected when choosing their degree programme," adds Prem, who also teaches ethics to future developers. And that although AI contains a lot of social science, such as communication science, cognitive sciences, computational linguistics or interface design, says Katja Mayer from the Department of Science and Technology Studies, "Unfortunately, their critical dimensions are often not considered enough in technology development. If we want to understand AI as a socio-technology, it is essential to increase the inclusion or visibility of the critical social sciences."
Exchanging perspectives for gender-fair translation machines
With this aim in mind, computer linguist Dagmar Gromann and a cross-institutional team have brought together non-binary persons, translators and developers of machine translation systems in a three-day workshop. They evaluated the needs of the different groups of people and how gender-sensitive machine translation can work (to the guidelines on gender-sensitive language by the Ombud for Equal Treatment, in German). While representatives of the community of non-binary persons expressed a desire for respect and acceptance of diverse gender concepts, the representatives of the language industry called for standardisation and norms, which can be easily implemented, reports Gromann, professor at the Centre for Translation Studies.
"We finally agreed on a step-by-step model that works similar to a washing machine's quality label: The generic masculine or the German Binnen-I are highlighted in red, the level above is characterised by neutral formulations or the gender star, and the green area is characterised by gender-neutral language, so to speak, which takes all gender identities into account," says Gromann.
The model aims to provide clear application examples and explain how gender-sensitive language can be implemented by automatic means. "And because changes in our language do not happen overnight, we need to offer several options. Companies or clients of translators can decide on a 'level' and the machine provides a translation that consistently uses the relevant variant."
How about debiasing?
Debiasing is the term used to describe methods and strategies for decision-making that help reduce prejudices and biased judgements – biases – in neural language models and raise awareness of these issues. Debiasing of AI systems has become a separate field of research, but biases are deeply entrenched and firmly anchored in our language: Until now, models cannot speak respectfully about all identities while being performant at the same time, explains Dagmar Gromann. One strategy is to draw the users' attention to the bias, "but this shifts responsibility onto the users instead of fixing the actual problem," adds Prem.
Speaking of decisions: The best and most relevant AI models on the market come from non-academic institutions, i.e. private companies. These companies make decisions about the (moral) design of AI systems themselves. For example, with the emergence of ChatGPT and Co., violence, child pornography or medical advice were put on the ‘red list’. "However, these topics are complex and there are contexts in which we may need them," Prem explains, "A counselling tool should be able to address violence; when I am working on a novel, I want to also write creepy passages; when training future doctors, I may need images that show evidence of violence."
But machines still find it difficult to act according to context – "Here, the human decision is still the gold standard.” The problem is: Red-list content in the training data for AI-powered systems is often manually sorted out by people in low-wage countries. The people responsible for sorting out this often traumatic content do not receive any psychological support, criticises Prem.
AI systems hold up a digital mirror that reflects our history, our being. And sometimes we realise that we do not like this – when we are misogynistic or right-wing extremist or in some other way awful. But once we see these circumstances mirrored in a computer program, we can firstly analyse them and secondly improve them.Erich Prem
Cooperating to regulate AI
Artificial intelligences are determined by local conditions as well as by global infrastructures. To make the use of AI as fair as possible, it is necessary to pursue transdisciplinary and also multidimensional approaches, which include ethical, regulatory, technical as well as knowledge- and education-related measures, explains Mayer, advocating "clear guidelines and standards that reflect the dynamic development of the technologies themselves and their abilities to learn." The EU is working intensively to develop an AI regulation and the UN has also already set up a working group focusing on this issue. It remains to be seen whether regulation will make AI systems and ultimately our society fairer. Or according to ChatGPT: The issue is complex. (hm)
With her research on gender-fair language and language technology, she aims to contribute to a respectful approach to different gender models.
How much and what kind of openness is needed to ensure that machine learning remains controllable and manageable, in the interests of the common good in the present and in the future? She is currently discussing these and similar issues with the aim of improving open science practices and policies.
Prem is currently working on ethical and epistemological questions of artificial intelligence. As a consultant, he supports (public) institutions with regard to AI ethics.
- Centre for Translation Studies
- Website of Dagmar Gromann
- Department of Science and Technology Studies
- Website of Katja Mayer
- IMoox course "Digital life" with Katja Mayer and others
- Department of Philosophy
- Website of Erich Prem
- Reading recommenndation: Our digital mirror by Erich Prem, published in Perspectives on Digital Humanities
- University of Vienna researchers on the semester question: Do we know what AI will know?