AI detects river pollution
Pollution in our rivers comes from a variety of sources, including pesticides and fertilizers used in agriculture. Many industries use large amounts of river water for a number of purposes, such as cooling and cleaning, and then return the water to the river through water treatment plants. Associated with these treatment plants is the constant risk of chemical release into rivers. Chemicals, particularly micropollutants, have extremely harmful effects on both people and water habitats. Thus, identifying contaminants in rivers and determining their origin is critical. Until now, the technologies have been both too costly and too slow to identify micropollutants in near real-time.
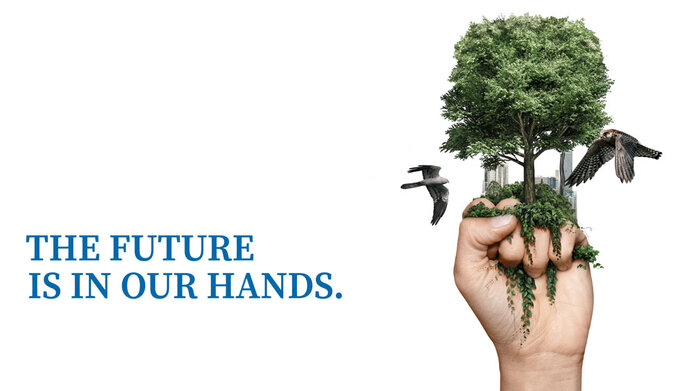
Environment and Climate Research Hub (ECH)
Atakan Aral is a member of the Environment and Climate Research Hub (ECH), the new multidisciplinary research network within the University of Vienna. It is dedicated to connecting researchers addressing environment, climate, and sustainability from different academic viewpoints. More about the objectives of the network.
Currently, the ECH has 65 members from different faculties and departments of the University of Vienna. All of them carry out research in the field of environment and climate.
Fast detection is crucial
The goal of the SWAIN project, led by Atakan Aral from the Research Group Scientific Computing at the University of Vienna, is to create an early warning system for micropollutant spills in rivers, using artificial intelligence. "Earlier in my research, I focused on urban areas like smart cities and smart buildings. 2019 was my turning point, when I witnessed the disaster in Poland, where a huge amount of wastewater spilled into the Vistula River. Tons of nitrogen, and phosphorus contaminated the water and this continued for days. I was astonished that it took them five days to detect the issue", says Aral. This is also the case with many other disasters – such dangerous events often go unnoticed for a long time. They are only discovered after a mass death of fish or mussels.
"As someone who works on sensors and distributed computing systems, I was curious why there is no continuous monitoring system or some kind of early warning system that could alleviate such tragedies. This is where the initial idea came from", explains Aral. He started collaborating with people from environmental sciences and international experts from Austria, Finland, Switzerland, and Turkey. Together they created the international project called SWAIN with the ultimate goal to create and provide the first artificial intelligence-based early warning and prediction system for agricultural, industrial, and residential pollution in European waterways. The system will locate the source of the pollution within minutes and provide a near real-time response.
Predictions by AI
Aral and his team use artificial intelligence in the form of a machine learning model combined with sensors that continuously collect different kinds of environmental data in rivers. The AI will compare these data to historical ones and further make predictions. "The main novelty of our systems is that we are not only able to detect pollutants, but we can also backtrack them in the river network and detect the origin of the pollution. Within minutes after a spill, the system can identify the origin by analysing water flow patterns", Aral points out.
Their machine learning approach is based on a technique known as Graph Neural Networks. It processes data by looking at it as a graph or a network. This works well for river basins with their many branching tributaries and channels. To train the model, the researchers use data such as the speed of the water, the shape of the river, and the amount of rain.
The system will be validated in the Ergene River in Turkey, which is well known as one of the most polluted rivers in Europe. The upper reaches of the Ergene River are home to many industries that use the river's water, while its lower reaches are set in some of the largest agricultural areas in Turkey, which use the water for irrigation.
To generalise their results and show that they were not specific to a certain river, the researchers added another river system to the project: the Kokemäenjoki river in Finland, located in a completely different environment and ecosystem. The city of Turku uses groundwater from the waters of the Kokemäenjoki. Pollutants from the river upstream can travel to the water intake site in 20 minutes to 1 or 2 hours depending on the flow. Therefore, timely detection of the pollutants would let officials stop water intake before utility water is contaminated.
Environmental monitoring by the "Internet of Things"
The Internet of Things (IoT) is another technology used in this project. The main benefit is that it replaces manual data collection, which is both costly and slow. "In our case, we have sensors all around the river that collect data and are connected to the internet. They share all the information with us in real-time", says Aral. IoT, however, is dependent on network connectivity, and as the study areas are in rural and remote areas, the team faces significant challenges concerning connectivity. "Another limitation of IoT is that we have to collect data from many locations. For example, in one system, we had 70 collection points. This has some environmental impact because these are electronic circuits that are left in the environment. It's difficult, maybe impossible, to go back and collect them later. They are battery-powered and could be harmful to the environment," Aral explains.
As a result, Aral aimed to reduce the number of IoT devices, while at the same time, collecting equal-quality data. The solution to this was to eliminate any sampling points that were unnecessary or offered insufficient evidence and to optimise sensor location to maximise information. In the Ergene River, the project started with 70 sampling locations. These were now reduced to 15. "This was recently the main breakthrough of the project. We managed to reduce network utilization and increase energy efficiency. That is an enormous progress", says Aral.
The next phase will be to create a self-sustaining ecosystem of computing, without the need for batteries, requiring little maintenance, and generating data without harming the environment. For that, solar energy or the kinetic force of the river flow will be used.
The SWAIN Project will play a significant role in preserving natural water ecosystems and protecting public health. "We not only contribute new findings to the academic field but also provide real-world implications, like preventing ecological disasters and ensuring safe drinking water", says the computer scientist.
What is the Internet of Things?
The Internet of Things (IoT) links physical objects to the virtual world. Smart devices and machines are connected to each other via a network. Through their interaction, they gather and analyse data about their immediate surroundings. This enables them to carry out specific functions. For instance, an IoT device can adjust the heating when a sensor detects the outside temperature. This is achieved by interacting with networked components, such as microcontrollers, sensors, and actuators - which convert electrical impulses into mechanical variables such as pressure, motion, temperature, or others.
He is the International Coordinator of the SWAIN project and Principal Investigator of the TROCI project (starting in 2024), supported by EU CHIST-ERA activity and FWF. He is a member of the Environment and Climate Research Hub.